Book Appointment Now
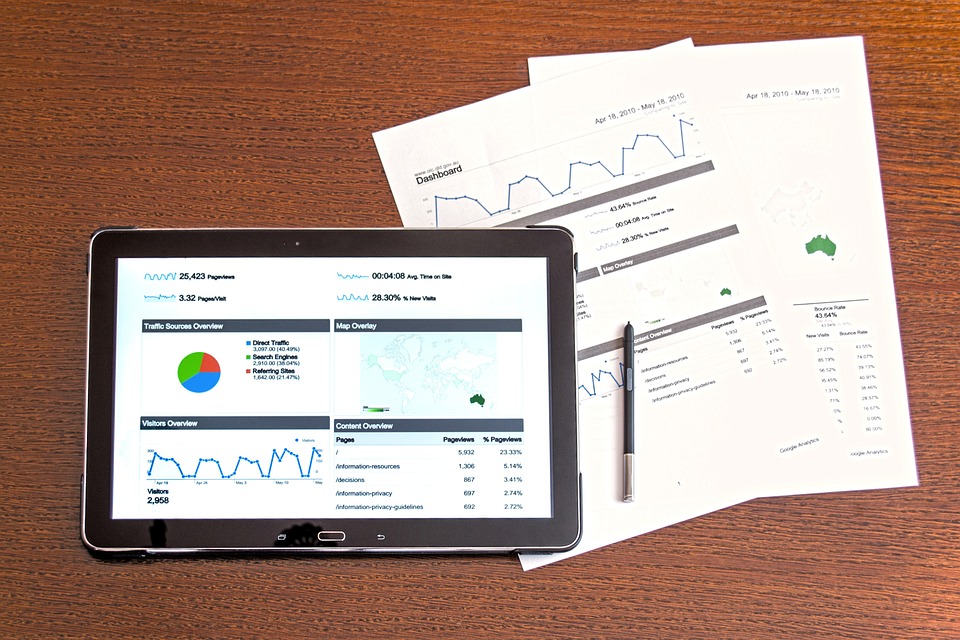
Data-Driven Staffing Models
In today’s healthcare environment, data-driven staffing models are becoming essential for managing nurse workloads, preventing burnout, and enhancing patient care. Healthcare institutions are increasingly relying on advanced data analytics to balance nurse staffing, streamline shift planning, and maintain optimal nurse-to-patient ratios. By using real-time data, hospitals and clinics can ensure that their workforce is efficiently allocated and that nurses have the support they need to thrive in their roles.
Get a nursing paper help with your data-driven staffing model assignment
Order Custom Nursing Paper
How Data-Driven Staffing Models Improve Nurse Workload Management
With nurse staffing optimization, healthcare institutions can minimize the risk of nurse burnout by ensuring that staffing levels match patient demand. These models use a variety of data points, including patient acuity, nurse experience, and historical workload trends, to develop shift schedules and allocate nurses more effectively. By analyzing this data, healthcare administrators can avoid understaffing or overstaffing, both of which contribute to nurse stress and burnout.
Benefits of Data-Driven Staffing for Shift Planning
- Real-Time Adjustments: With data analytics, healthcare facilities can make real-time adjustments to staffing plans, responding to fluctuations in patient volumes and acuity levels.
- Predictive Analytics: By forecasting patient needs and nurse availability, data-driven models help plan shifts and anticipate potential staffing shortages or surpluses.
- Optimized Nurse-to-Patient Ratios: Optimal nurse-to-patient ratios are crucial for ensuring that each nurse can provide high-quality care. Data-driven models enable institutions to maintain the right balance, preventing burnout and enhancing patient safety.
- Improved Nurse Retention: By aligning workloads with nurse preferences and availability, nurse retention strategies are strengthened, leading to higher job satisfaction and lower turnover rates.
- Cost-Efficiency: Data-driven staffing reduces costs associated with overstaffing, underutilization, and overtime, creating a more efficient healthcare workforce.
How Data-Driven Staffing Addresses Nurse Burnout
Nurse burnout has become a significant concern in healthcare settings, often leading to decreased job satisfaction, higher turnover rates, and compromised patient care. By implementing workload management strategies through data analytics, healthcare institutions can:
- Monitor workload trends and adjust nurse assignments accordingly.
- Identify signs of burnout early and take preventive actions, such as adjusting shifts or offering additional support to overburdened nurses.
- Support work-life balance by utilizing data to schedule shifts that accommodate nurses’ preferences, reducing stress and fostering job satisfaction.
Key Components of Effective Data-Driven Staffing Models
- Patient Acuity: Understanding the severity of patients’ conditions helps determine the number of nurses required per shift.
- Nurse Experience and Skills: Data analytics account for the skill set and experience level of nurses, ensuring the right fit for the level of care required.
- Historical Data: By examining past trends in patient volume and nurse workload, healthcare institutions can predict future staffing needs.
- Real-Time Monitoring: Continuous data collection during shifts ensures that staffing levels are adjusted dynamically to meet real-time needs.
The Future of Data-Driven Staffing in Healthcare
As healthcare systems continue to adopt new technologies, data-driven staffing models will only become more sophisticated. Artificial intelligence (AI) and machine learning will further enhance predictive analytics, providing even more precise staffing forecasts. By continuously refining these models, healthcare institutions can maintain efficient staffing, reduce nurse burnout prevention, and improve overall patient outcomes.
The use of data-driven staffing models is transforming the way healthcare institutions manage nurse workloads and prevent burnout. By incorporating data analytics into shift planning and staffing decisions, healthcare organizations can ensure optimal nurse-to-patient ratios, improve nurse satisfaction, and create a more efficient workforce. As data analytics technologies continue to evolve, so too will the ability to create balanced, sustainable staffing solutions that benefit both nurses and patients.