Book Appointment Now
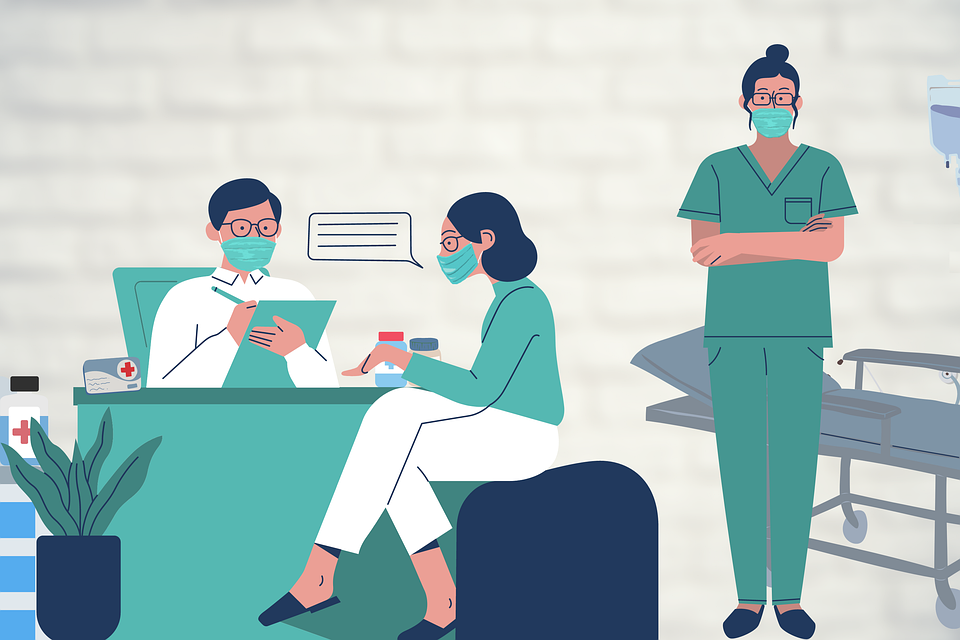
Adapting the Health Belief Model for Modern Public Health Challenges
The Health Belief Model (HBM) has long been a cornerstone of behavioral science, offering a framework to understand and predict health-related behaviors. Developed in the 1950s by social psychologists Hochbaum, Rosenstock, and Kegels, the HBM was initially used to explain low participation in tuberculosis screening programs. Since then, it has been applied across diverse health domains, including chronic disease prevention, vaccination campaigns, and health promotion. In today’s rapidly evolving public health landscape, marked by digital innovation, misinformation, and global health inequities, the HBM retains its relevance but requires adaptation to meet new challenges. This essay explores how the HBM can be integrated with digital technologies, address the influence of misinformation, and adapt to cultural and socioeconomic diversity to remain a powerful tool in public health.
Get a custom paper help about the Health Belief Model (HBM)
Order Custom Nursing Paper
Revisiting the Constructs of the Health Belief Model
The HBM posits that health behaviors are influenced by six primary constructs: perceived susceptibility, perceived severity, perceived benefits, perceived barriers, cues to action, and self-efficacy. These constructs collectively explain why individuals adopt or reject health-related behaviors. Perceived susceptibility and severity relate to an individual’s belief about their risk of experiencing a health condition and its seriousness. Perceived benefits and barriers weigh the positive outcomes of a health action against obstacles like cost or inconvenience. Cues to action serve as triggers for behavior change, while self-efficacy reflects confidence in one’s ability to perform the action (Glanz et al., 2015).
While these constructs remain foundational, their application requires a nuanced understanding of how modern challenges—such as misinformation and digital health technologies—intersect with individual decision-making processes. By addressing these intersections, public health practitioners can leverage the HBM to create tailored, culturally sensitive, and technology-driven interventions.
Leveraging Digital Health Tools to Enhance HBM Constructs
Digital health technologies, including mobile health (mHealth) applications, wearable devices, and telehealth platforms, have revolutionized the delivery of health information and services. These tools align seamlessly with the HBM by reinforcing its constructs in personalized and scalable ways. For instance, fitness trackers like Fitbit or smartwatches provide real-time feedback, serving as cues to action that prompt users to meet activity goals. They also increase self-efficacy by gamifying health behaviors, making users feel empowered to achieve small, incremental milestones (Maher et al., 2016).
Similarly, mHealth applications address perceived susceptibility and severity by providing tailored risk assessments and educational content. For example, apps designed for diabetes management can deliver personalized messages about blood sugar levels, emphasizing the importance of medication adherence or dietary modifications to prevent complications. By highlighting perceived benefits—such as reduced risk of complications—and minimizing perceived barriers like access to information, these tools promote sustained behavior change.
However, the effectiveness of digital health tools depends on their accessibility and usability, particularly among older adults or those with low technological literacy. Addressing these barriers is critical to ensuring equitable health outcomes and maximizing the HBM’s applicability in digital health interventions.
Combatting Misinformation and Vaccine Hesitancy
The proliferation of misinformation, particularly on social media, poses a significant threat to public health. Vaccine hesitancy, fueled by false claims about vaccine safety or efficacy, highlights the importance of using HBM constructs to counteract misinformation. For example, public health campaigns that emphasize perceived susceptibility and severity of vaccine-preventable diseases, such as COVID-19 or measles, can help individuals understand their risk of infection and the potential consequences of inaction (Betsch et al., 2020).
To enhance perceived benefits, messaging should focus on the collective impact of vaccination, such as achieving herd immunity or protecting vulnerable populations. Addressing perceived barriers, such as concerns about vaccine side effects, requires clear, evidence-based communication from trusted sources. Digital platforms can play a dual role in disseminating accurate information and identifying misinformation, leveraging algorithms to amplify credible content and reduce the visibility of false claims.
An example of HBM-informed communication is the World Health Organization’s (WHO) efforts to provide real-time updates on vaccine safety through its official website and mobile apps. By coupling these initiatives with targeted social media campaigns that resonate with specific cultural and demographic groups, the HBM can guide the design of interventions that counter misinformation and promote vaccine uptake.
Adapting the HBM to Cultural and Socioeconomic Diversity
Health behaviors are profoundly influenced by cultural beliefs, socioeconomic conditions, and access to resources. While the HBM provides a universal framework, its application must be tailored to account for these contextual factors. For instance, perceived barriers may differ significantly between populations in high-income and low-income settings. In resource-constrained environments, barriers such as transportation costs or lack of healthcare facilities may outweigh perceived benefits, even when individuals recognize the severity of their health condition (Nutbeam et al., 2010).
Cultural norms also shape perceptions of susceptibility, severity, and self-efficacy. In some cultures, fatalistic beliefs about illness may diminish individuals’ motivation to seek preventive care, while strong family or community ties can serve as powerful cues to action. Public health interventions that leverage community networks, traditional healers, or religious leaders as messengers can enhance the cultural relevance and impact of HBM-based strategies.
A successful example of culturally adapted HBM application is the promotion of maternal health interventions in rural India. By engaging local health workers to address perceived barriers, such as stigma surrounding antenatal care, and using culturally resonant messaging to emphasize perceived benefits, these programs have significantly improved maternal health outcomes (Singh et al., 2017).
Future Directions: HBM and Artificial Intelligence
The integration of artificial intelligence (AI) into public health offers exciting opportunities to expand the reach and precision of HBM-based interventions. AI-powered tools can analyze large datasets to predict health behaviors, tailoring interventions to individual needs. For instance, AI algorithms could identify individuals at high risk for chronic diseases based on their demographic and behavioral data, delivering personalized messages to enhance their perceived susceptibility and self-efficacy.
Additionally, AI chatbots and virtual health assistants can provide real-time responses to health queries, addressing perceived barriers such as lack of access to healthcare professionals. These tools can also reinforce cues to action through reminders and follow-up notifications, ensuring sustained engagement with health behaviors. However, ethical considerations, such as data privacy and algorithmic bias, must be carefully managed to ensure that AI-driven interventions promote equitable health outcomes.
Conclusion
The Health Belief Model remains a valuable framework for understanding and influencing health behaviors, but its application must evolve to address modern public health challenges. By integrating digital health technologies, countering misinformation, and adapting interventions to cultural and socioeconomic contexts, the HBM can retain its relevance in an increasingly complex healthcare landscape. Innovations such as AI and personalized health tools further enhance the model’s potential, enabling public health practitioners to design targeted, impactful interventions. As the global health landscape continues to change, the HBM offers a versatile and evidence-based foundation for promoting healthier behaviors and improving population health.
References
- Betsch, C., Schmid, P., Heinemeier, D., Korn, L., Holtmann, C., & Böhm, R. (2020). Beyond confidence: Development of a measure assessing the 5C psychological antecedents of vaccination. PLoS ONE, 13(12), e0208601. https://doi.org/10.1371/journal.pone.0208601
- Glanz, K., Rimer, B. K., & Viswanath, K. (2015). Health Behavior: Theory, Research, and Practice (5th ed.). Jossey-Bass.
- Maher, C., Ryan, J., Ambrosi, C., & Edney, S. (2016). Users’ experiences of wearable activity trackers: A cross-sectional study. BMC Public Health, 16(1), 780. https://doi.org/10.1186/s12889-016-3436-8
- Nutbeam, D., Harris, E., & Wise, M. (2010). Theory in a Nutshell: A Practical Guide to Health Promotion Theories (3rd ed.). McGraw-Hill Education.
- Singh, P. K., Rai, R. K., & Singh, L. (2017). Examining the effect of household wealth and migration status on safe delivery care in India, 1992–2006. PLoS ONE, 7(8), e3130. https://doi.org/10.1371/journal.pone.003130
- Zhao, Z., & Cui, Q. (2020). Artificial intelligence in healthcare: Big data-based decision-making systems. Journal of Healthcare Informatics Research, 4(1), 3-12. https://doi.org/10.1007/s41666-019-00093-7