Book Appointment Now
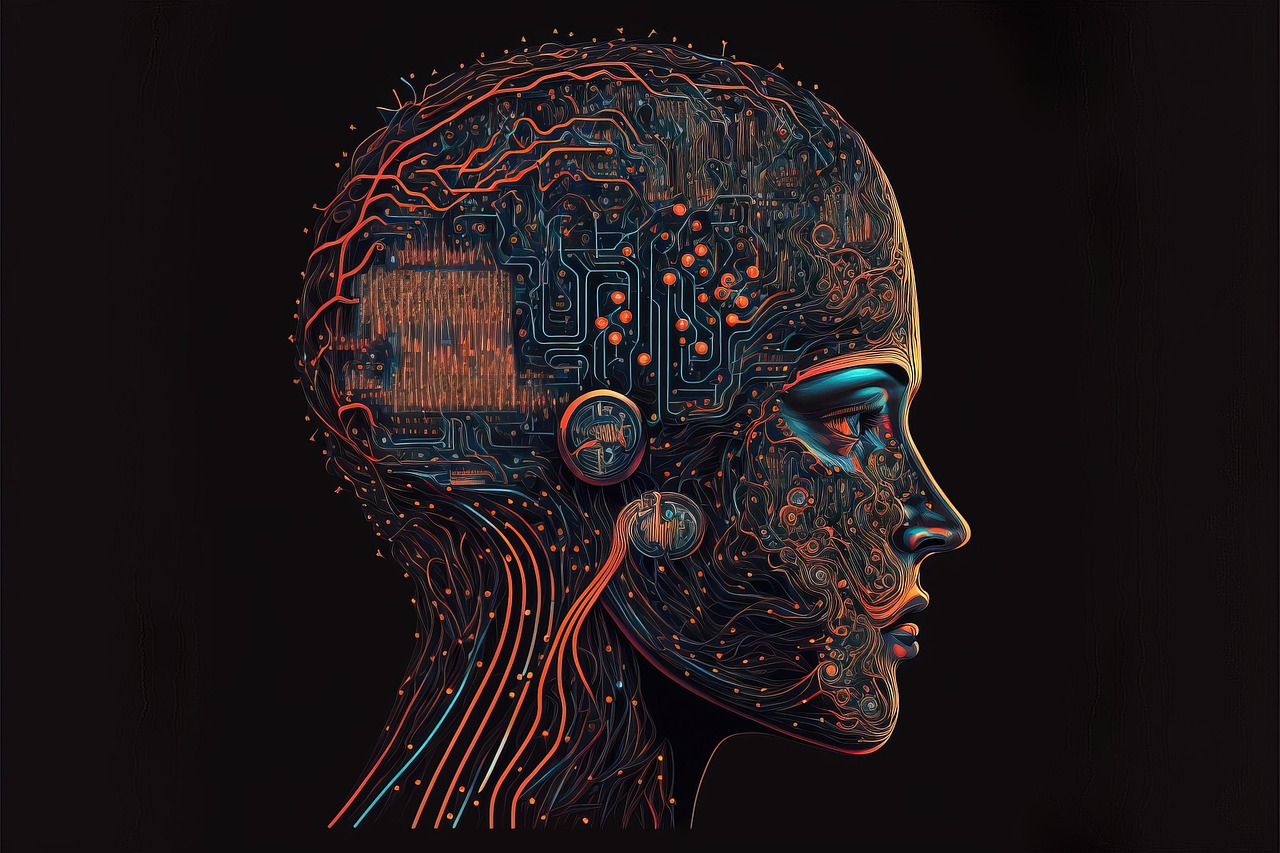
Bias in AI Algorithms in Nursing: How It Affects Nursing Care Delivery
In recent years, artificial intelligence (AI) has emerged as a transformative force in healthcare, offering unprecedented opportunities to enhance efficiency, accuracy, and patient outcomes. From diagnostics to personalized care planning, AI is increasingly embedded in clinical workflows—including nursing care delivery. However, as this technology becomes more integral to nursing practice, a critical issue demands attention: bias in AI algorithms in nursing. These biases—often rooted in historical data disparities, systemic inequalities, and limited model transparency—can undermine the very goals AI aims to achieve. When unchecked, they risk reinforcing existing healthcare disparities, skewing clinical decision-making, and compromising the ethical obligations of nursing professionals. Understanding the sources, manifestations, and consequences of this bias is essential to ensure that AI becomes a tool for inclusive and equitable care rather than a contributor to inequality.
Count on our nursing experts to deliver 100% custom paper about bias in AI algorithms in nursing
Write my nursing essay
Understanding AI Bias in Healthcare
Bias in AI arises when an algorithm produces systematically prejudiced outcomes due to erroneous assumptions in its data or design. In healthcare, this could mean anything from unequal diagnostic accuracy across demographic groups to discriminatory treatment recommendations (Obermeyer et al., 2019). When such algorithms are deployed in nursing care—be it for triaging patients, monitoring vital signs, or recommending interventions—their biases can directly influence the decisions and actions of nurses.
Sources of AI Bias in Nursing Applications
There are several key sources of bias that affect AI algorithms used in nursing:
-
Data Imbalance: AI systems are only as good as the data they are trained on. If historical healthcare data lacks diversity—such as underrepresentation of minority populations—the algorithm may not generalize well to all patients (Wiens et al., 2020).
-
Human Bias in Labeling: If clinicians’ judgments are used to train models, existing prejudices in human decision-making can be baked into the algorithm. For example, if certain symptoms are historically overlooked in women or racial minorities, the AI will replicate this oversight.
-
Design Bias: The people who design and train algorithms may unintentionally encode their own biases, particularly if they lack awareness of social determinants of health or real-world nursing workflows.
-
Deployment Context: Even well-designed models can produce biased results if applied in settings for which they weren’t intended. For example, a model trained in a high-income hospital may perform poorly in a rural clinic with different patient demographics.
Impacts on Nursing Care Delivery
Nursing is a frontline profession where timely, accurate, and compassionate care is essential. AI bias, if unaddressed, can affect this in several ways:
1. Inaccurate Risk Stratification
Many nursing workflows now incorporate AI-powered tools for patient risk assessment. A biased algorithm may overestimate or underestimate a patient’s risk depending on race, gender, or socioeconomic status, leading to misallocation of nursing resources or failure to escalate care when necessary.
2. Worsening Health Disparities
If AI tools reinforce systemic biases, vulnerable populations may receive substandard care. A well-documented case by Obermeyer et al. (2019) showed how a commercial algorithm used in U.S. hospitals underestimated the health needs of Black patients compared to white patients with the same level of chronic illness.
3. Erosion of Trust in Technology
Nurses who detect discrepancies between AI recommendations and clinical judgment may become skeptical of such tools. This lack of trust can hinder adoption and integration of potentially beneficial technologies.
4. Compromised Ethical Standards
Nurses are ethically obligated to provide equitable care. Using biased AI tools could place them in ethical conflicts, especially if they’re forced to rely on systems that systematically disadvantage certain patients.
Addressing Bias: A Nursing Informatics Perspective
To mitigate the negative effects of AI bias in nursing care, a multifaceted approach is essential:
1. Inclusive Data Collection
Healthcare institutions must prioritize the collection of diverse, high-quality data that accurately represents the population. This includes data stratified by race, gender, age, and socioeconomic status.
2. Bias Auditing and Transparency
Before deployment, AI tools should undergo rigorous bias testing. Independent audits can help identify potential issues. Transparency in how algorithms work allows nurses and other clinicians to understand the rationale behind decisions.
3. Training and Education
Nursing education should include modules on AI literacy, enabling nurses to critically assess AI tools. Understanding where biases come from and how to spot them will empower nurses to use these tools responsibly.
4. Interdisciplinary Design Teams
AI development should involve nurses, ethicists, and community representatives—not just data scientists. This inclusive approach ensures that models consider practical realities and ethical concerns.
The Role of Policy and Regulation
Governments and healthcare regulators must step in to establish standards for ethical AI use in nursing care. Frameworks such as the EU’s AI Act or guidelines from professional bodies like the American Nurses Association can provide guidance on data governance, accountability, and bias mitigation.
The rise of AI in healthcare is reshaping how nursing care is delivered, but it also presents significant ethical and practical challenges. Bias in AI algorithms in nursing is not a theoretical concern—it has real-world implications that can jeopardize patient safety, worsen health disparities, and place nurses in difficult ethical positions. As frontline caregivers, nurses must be empowered with both the knowledge and the tools to critically engage with AI systems. At the same time, healthcare institutions, technologists, and policymakers must work collaboratively to ensure that AI tools are designed with equity, inclusivity, and transparency at their core. Only by confronting and correcting algorithmic bias can we ensure that AI fulfills its promise of enhancing—not hindering—nursing care for all.
References
-
Obermeyer, Z., Powers, B., Vogeli, C., & Mullainathan, S. (2019). Dissecting racial bias in an algorithm used to manage the health of populations. Science, 366(6464), 447–453. https://doi.org/10.1126/science.aax2342
-
Wiens, J., Saria, S., Sendak, M., Ghassemi, M., Liu, V. X., Doshi-Velez, F., Jung, K., Heller, K., Kale, D., Saeed, M., Ossorio, P. N., & Thadaney Israni, S. (2020). Do no harm: A roadmap for responsible machine learning for health care. Nature Medicine, 25, 1337–1340. https://doi.org/10.1038/s41591-019-0548-6