Book Appointment Now
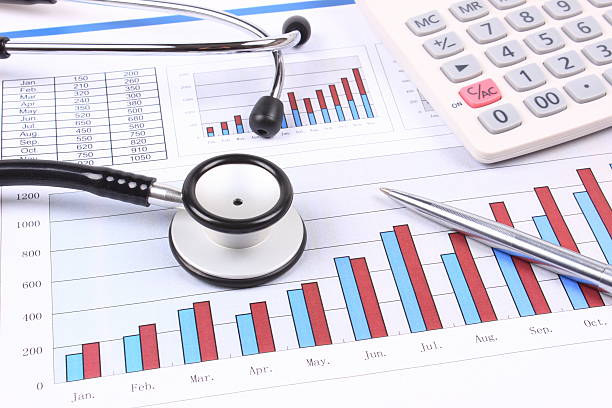
Reducing Hospital Readmissions Through Data-Driven Interventions
Hospital readmissions are a significant concern for healthcare systems globally, particularly for patients managing chronic diseases. Reducing hospital readmissions not only improves patient outcomes but also reduces healthcare costs and increases hospital efficiency. Data-driven interventions play a critical role in preventing unnecessary readmissions by using predictive analytics, improving care coordination, and enhancing patient education and follow-up care.
Get a custom nursing paper on reducing hospital readmissions through data-driven interventions assignment
Order Custom Nursing Paper
How Data-Driven Decision-Making Reduces Hospital Readmissions
Data-driven decision-making allows healthcare providers to analyze patient data, identify risk factors for readmission, and implement targeted interventions to prevent avoidable hospital stays. Here are some key strategies:
- Predictive Analytics to Identify High-Risk Patients
By analyzing patient data, including medical history, comorbidities, and social factors, hospitals can identify patients at high risk of readmission. Predictive analytics can flag these patients, allowing healthcare providers to prioritize follow-up care and interventions.- Case Study: The use of predictive modeling at a major hospital system helped reduce heart failure readmissions by 25% within one year. By analyzing a range of variables, including past hospitalizations and medication adherence, the hospital team could identify high-risk patients and intervene early with tailored care plans.
- Personalized Chronic Disease Management Plans
Data collection enables healthcare providers to create personalized care plans for patients with chronic diseases like diabetes, heart disease, and COPD (chronic obstructive pulmonary disease). Regular monitoring and data collection help providers track patient progress and make adjustments to treatment plans as necessary.- Case Study: At an urban health center, a diabetes management program that utilized data from glucose monitoring devices and electronic health records (EHR) reduced readmission rates by 30%. By continuously monitoring patients’ glucose levels and adjusting medication doses in real-time, healthcare providers were able to prevent complications that would lead to hospitalization.
- Optimized Discharge Planning and Follow-Up Care
Effective discharge planning is critical to preventing readmissions. Data-driven approaches can help ensure patients understand their care instructions, have access to medications, and are scheduled for follow-up appointments. Real-time data helps healthcare providers anticipate potential issues that could arise post-discharge, such as complications or lack of resources.- Case Study: A hospital in Massachusetts implemented a discharge planning intervention that included follow-up phone calls, reminders for outpatient appointments, and home health visits. The program led to a 15% reduction in readmissions for patients with congestive heart failure (CHF) by addressing post-discharge issues before they became critical.
- Improved Care Coordination Between Providers
Effective communication between primary care providers, specialists, and hospital teams is essential in reducing readmissions. Data-sharing platforms and EHR systems enable better coordination and ensure that patient information is easily accessible across the continuum of care.- Case Study: A healthcare system in California integrated EHRs across primary care, emergency departments, and hospitals, which allowed for seamless coordination of care. This effort helped reduce readmission rates for patients with chronic conditions like asthma by 20%, as all providers had up-to-date information and could provide timely interventions.
- Patient Education and Self-Management
Data-driven interventions also focus on empowering patients to manage their own health. By utilizing health apps and wearable devices, patients can track their vital signs and symptoms, allowing them to detect early signs of a health issue and take preventive measures.- Case Study: A health network introduced a telehealth platform where patients with chronic respiratory conditions could input data from home spirometry tests. Nurses and doctors could monitor patients remotely, adjust medications as needed, and provide timely advice. This remote management approach resulted in a 10% reduction in readmission rates for COPD patients.
- Utilizing Social Determinants of Health (SDOH) Data
Social factors, such as access to transportation, housing stability, and social support, can significantly impact a patient’s likelihood of readmission. Data collection systems that include SDOH can help healthcare teams address these barriers to care and provide more holistic treatment.- Case Study: A hospital in Ohio used data on patients’ social circumstances, such as housing instability and lack of access to transportation, to identify those at risk of readmission. By providing these patients with transportation to follow-up appointments and connecting them to community resources, readmission rates decreased by 18%.
The Role of Predictive Analytics in Reducing Readmission Risk
Predictive analytics plays a central role in identifying patients who are at high risk of readmission. By analyzing historical patient data, demographic information, and real-time health metrics, healthcare providers can forecast potential complications and intervene before a patient needs to be readmitted.
- Risk Scoring Systems: Healthcare systems often implement risk-scoring algorithms based on predictive analytics to assess a patient’s likelihood of readmission. These systems use a combination of patient data, including diagnosis codes, medical history, and hospital stay length, to assign risk scores that guide care management strategies.
- Real-Time Monitoring Tools: For patients with chronic conditions, wearable devices and remote monitoring systems allow healthcare teams to track vital signs, medication adherence, and other health metrics. If any signs of deterioration are detected, timely interventions can be made to prevent readmissions.
Long-Term Impact of Data-Driven Interventions
The long-term benefits of data-driven interventions go beyond reducing readmissions. These strategies contribute to:
- Improved patient satisfaction as patients receive more personalized, consistent, and comprehensive care.
- Lower healthcare costs by reducing unnecessary hospital stays and the need for costly emergency interventions.
- Better overall health outcomes, as patients with chronic conditions receive continuous care and preventive interventions, leading to improved quality of life.
Reducing hospital readmissions is a critical challenge for healthcare providers, especially in the management of chronic diseases. By leveraging data-driven interventions, hospitals and clinics can identify at-risk patients, optimize care plans, improve coordination, and provide better post-discharge support. Predictive analytics, personalized care, and real-time monitoring tools are all essential components of a strategy aimed at preventing readmissions. As healthcare continues to evolve, the integration of data into care management will remain a cornerstone of improving patient outcomes and reducing healthcare costs.
Also read:
- NSG 4064 Week 2 Assignment 2 COPD Readmission Interviews
- PHC 4305 Hospital Readmissions Reduction Program