Book Appointment Now
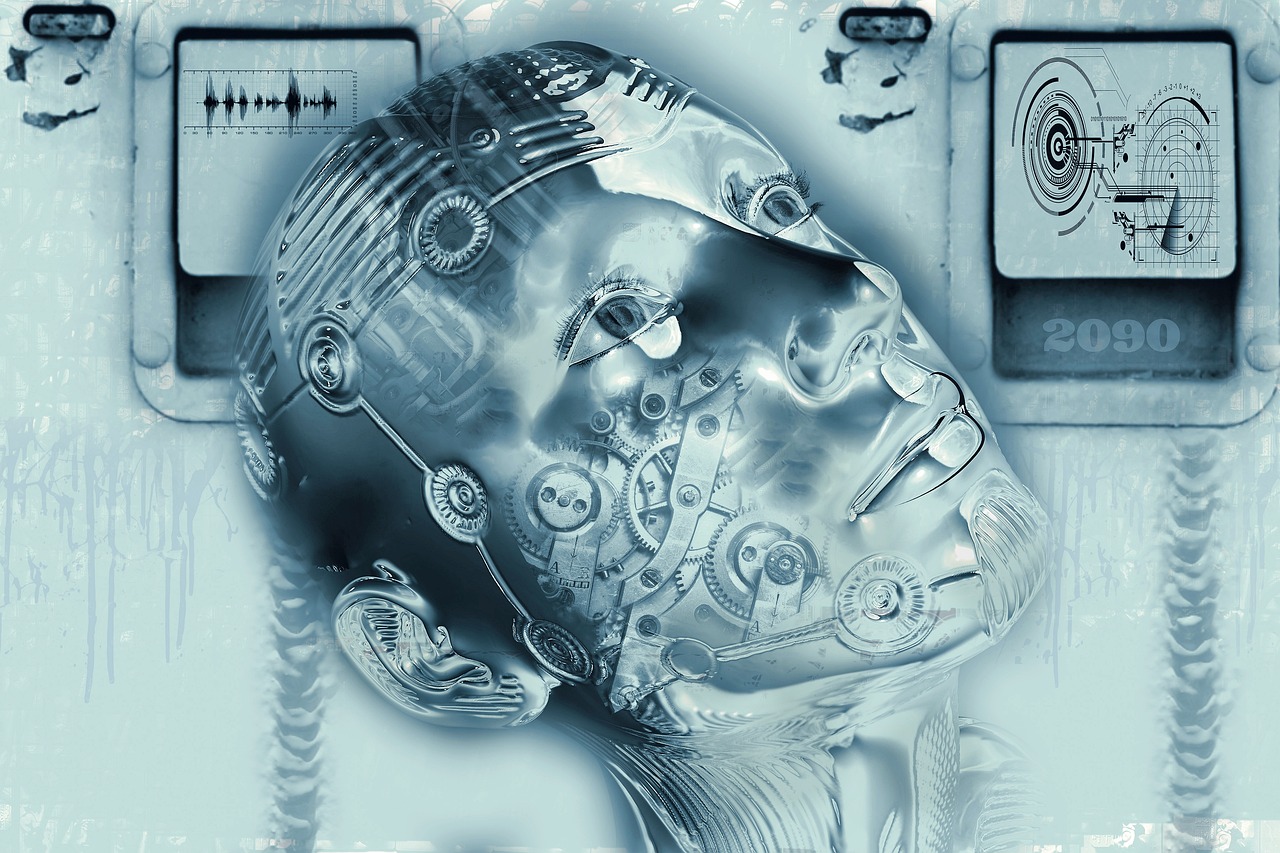
The Ethics of Artificial Intelligence in Patient Safety Monitoring
Artificial Intelligence in Patient Safety Monitoring represents a groundbreaking shift in modern healthcare, offering tools capable of predicting risks, preventing errors, and safeguarding patient well-being with unprecedented precision. From algorithms that detect early signs of sepsis to systems monitoring medication administration in real time, AI holds the potential to revolutionize how hospitals prevent harm and deliver care. However, as these technologies become integral to clinical decision-making, they also raise profound ethical questions. Concerns about algorithmic bias, transparency, accountability, and the erosion of human judgment challenge stakeholders to balance innovation with responsibility. This article examines the ethical landscape of AI-driven patient safety monitoring, exploring how healthcare systems can harness its benefits while upholding the core values of equity, trust, and patient-centered care.
1. The Promise of AI in Patient Safety
AI-driven tools are reshaping patient safety by enabling predictive analytics, error reduction, and workflow efficiency. For instance, predictive models can identify patients at risk of sepsis or cardiac arrest hours before symptoms manifest, allowing clinicians to intervene proactively. Real-time medication monitoring systems flag dosage errors or allergic reactions, minimizing preventable harm. Additionally, AI automates repetitive tasks such as continuous vital sign tracking, freeing healthcare providers to focus on complex decision-making. By aggregating and analyzing vast datasets, AI also uncovers patterns that might elude human observation, offering insights to refine care protocols. Despite these benefits, the ethical implications of delegating life-or-death decisions to algorithms demand rigorous scrutiny.
2. Ethical Concerns in AI-Driven Patient Safety
A. Transparency and Explainability
A central ethical challenge lies in the “black box” nature of many AI systems, where even developers struggle to explain how outputs are generated. Clinicians relying on AI for critical decisions—such as whether to escalate care or adjust treatment—require clear rationales to trust and validate recommendations. Opaque algorithms risk fostering blind reliance, potentially leading to inappropriate interventions or overlooked contextual factors, such as a patient’s unique preferences or socioeconomic barriers. To mitigate this, developers must prioritize “explainable AI” (XAI) models that provide interpretable decision pathways. Transparent documentation of data sources, algorithmic limitations, and potential biases is equally essential to build clinician and patient trust.
B. Bias and Equity
AI systems trained on biased or non-representative datasets risk perpetuating healthcare disparities. For example, an algorithm developed using data from predominantly white populations may fail to accurately detect sepsis in patients of color, delaying life-saving interventions. Similarly, tools optimized for cost reduction rather than patient outcomes might disproportionately harm underserved communities. Such biases undermine the ethical imperative of equitable care. Addressing this requires proactive measures: diversifying training datasets, auditing algorithms across demographic groups, and involving ethicists in the development process. Without these steps, AI risks entrenching systemic inequities under the guise of technological neutrality.
C. Accountability and Liability
Determining responsibility when AI tools err remains a legal and ethical quagmire. If an algorithm overlooks a critical medication interaction, who bears liability—the clinician who followed the alert, the hospital that adopted the tool, or the developer who designed it? Existing legal frameworks are ill-equipped to handle these scenarios, creating ambiguity that may discourage innovation or incentivize risk-averse practices. Clear guidelines for shared accountability are needed, balancing the responsibilities of developers, healthcare institutions, and end-users. Regulatory bodies like the FDA and WHO must collaborate with stakeholders to establish standards that protect patients while fostering ethical innovation.
D. Privacy and Data Security
AI’s reliance on vast amounts of patient data intensifies concerns about privacy and consent. Patients are often unaware their health information is used to train or refine AI models, raising questions about autonomy and transparency. Furthermore, vulnerabilities in AI systems—such as data breaches or unauthorized access—could expose sensitive records, eroding trust in healthcare institutions. Safeguarding privacy demands robust encryption, strict data anonymization protocols, and explicit patient consent processes. Policymakers must also ensure compliance with regulations like HIPAA and GDPR, which govern data protection but require updates to address AI-specific risks.
E. Human-AI Collaboration
While AI enhances efficiency, over-reliance on technology risks eroding clinical judgment and empathy. Clinicians may defer to algorithmic recommendations even when their intuition or experience suggests an alternative approach, potentially compromising patient-centered care. Patients, too, might feel alienated by interactions mediated through impersonal algorithms. Preserving the human element in healthcare requires designing AI as a decision-support tool rather than a replacement for clinician expertise. Training programs should emphasize critical evaluation of AI outputs, ensuring providers retain agency in final decisions.
3. Case Studies: Ethical Dilemmas in Action
Real-world examples highlight the stakes of unaddressed ethical flaws. The Epic Sepsis Model, a widely used AI tool, faced criticism for generating excessive false alarms, leading to alert fatigue and missed cases. Its proprietary design hindered independent validation, exemplifying the risks of opaque algorithms. Similarly, during the COVID-19 pandemic, studies revealed racial bias in pulse oximeters—devices that inform AI monitoring systems—which overestimated blood oxygen levels in darker-skinned patients, delaying critical treatment. These cases underscore the urgent need for ethical rigor in AI development and deployment.
4. Principles for Ethical AI Implementation
To align AI with patient safety goals, stakeholders must adopt frameworks grounded in fairness, transparency, accountability, privacy, and human-centered design. Fairness requires proactive mitigation of bias through diverse data and continuous auditing. Transparency mandates clear communication about how AI tools function, including limitations and potential conflicts of interest. Accountability hinges on defining roles for developers, providers, and institutions in addressing errors. Privacy protections must prioritize patient consent and data security. Finally, human-centered design ensures AI augments—rather than replaces—clinical expertise, preserving empathy and critical thinking in care delivery.
5. The Path Forward: Collaboration and Regulation
Ethical AI implementation demands collaboration across disciplines and sectors. Regulators must modernize policies to address AI-specific risks, as seen in the EU’s AI Act and the FDA’s evolving guidelines for AI-based medical devices. Interdisciplinary teams—including ethicists, clinicians, data scientists, and patients—should co-design systems to balance technical efficacy with ethical considerations. Hospitals must also invest in training programs that empower staff to critically engage with AI tools and report ethical concerns.
The integration of Artificial Intelligence in Patient Safety Monitoring marks a pivotal moment in healthcare—one where technology and ethics must evolve in tandem. While AI’s ability to predict complications and reduce errors is transformative, its ethical deployment demands rigorous safeguards against bias, opaque decision-making, and over-reliance on automation. Ensuring transparency, fostering equitable design, and preserving the clinician-patient relationship are non-negotiable priorities. As hospitals navigate this frontier, collaboration among developers, regulators, and healthcare providers will be critical to building systems that prioritize both innovation and integrity. Ultimately, the success of AI in patient safety hinges not just on its technical prowess, but on its ability to earn and maintain the trust of those it aims to protect: patients and the professionals who care for them. By grounding progress in ethical principles, healthcare can unlock AI’s full potential without compromising the humanity at the heart of medicine.