Book Appointment Now
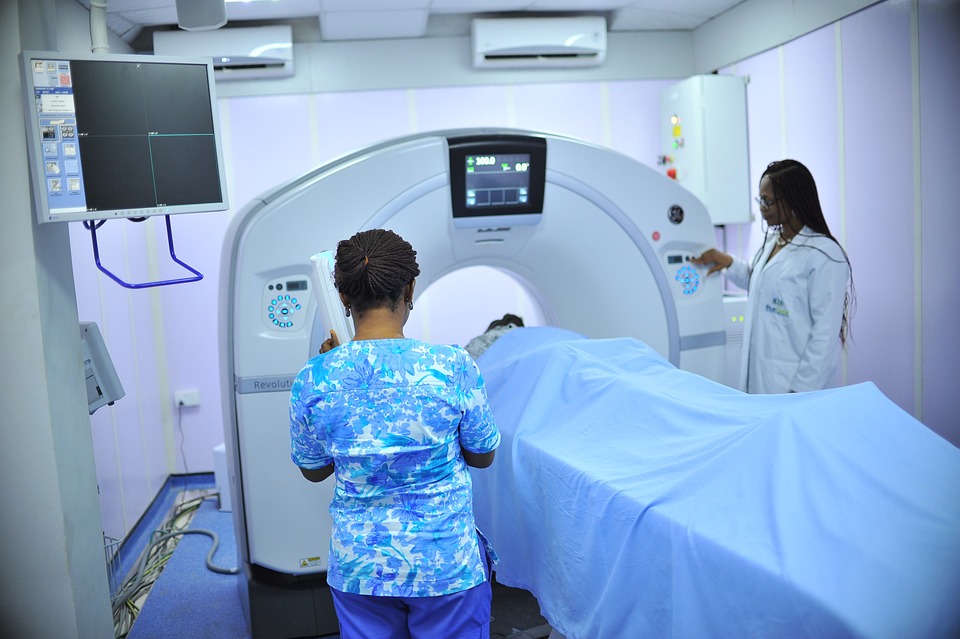
The Impact of Artificial Intelligence on Nursing Workflows
The integration of Artificial Intelligence (AI) into healthcare is transforming how nurses perform critical tasks, from patient care to administrative duties. As frontline caregivers, nurses often navigate a wide array of responsibilities, balancing patient monitoring, data analysis, decision-making, and documentation. AI technologies are stepping in to alleviate these demands, enabling nurses to focus more on direct patient interactions while improving the accuracy and efficiency of their work. By leveraging AI-powered tools, such as real-time monitoring systems, predictive analytics, and decision support platforms, nursing workflows are becoming increasingly data-driven and proactive. These technologies not only enhance clinical outcomes but also address long-standing challenges, such as alarm fatigue, information overload, and administrative burdens. However, the adoption of AI also raises critical questions about training, ethical considerations, and the evolving role of nurses in a technology-driven healthcare landscape. This paper explores The Impact of Artificial Intelligence on Nursing workflows with a detailed focus on its impact on patient monitoring, data analysis, and administrative efficiency.
Our nursing experts can deliver 100% custom paper about Impact of AI on nursing workflows according to your requirements.
Let us help you
1. Enhancing Patient Monitoring with AI Tools
Real-Time Vital Sign Monitoring
The role of AI in patient monitoring has gained prominence, particularly with the introduction of real-time vital sign tracking systems. Traditional monitoring systems require nurses to check vitals manually or rely on periodic updates from devices that may not capture critical changes promptly. AI-powered systems, such as wearable devices, enable continuous monitoring by collecting and analyzing data from patients in real time. Devices like Fitbit, Apple Watch, and specialized hospital-grade wearables are equipped with sensors that track heart rate, blood pressure, oxygen saturation, and even activity levels. Also read: TheFierceEMR and FierceHealthIT Narrated PowerPoint Presentation Topic: Patient Monitoring Technology
For example, EarlySense, an AI-driven monitoring platform, uses sensors placed under hospital beds to measure respiratory and heart rates continuously. A study by Ruppel et al. (2021) demonstrated that the use of such systems reduced the incidence of adverse events by 39%, as nurses were alerted to subtle changes in patients’ conditions well before critical thresholds were crossed. This proactive approach minimizes the risks associated with delayed interventions and allows nurses to allocate their attention more efficiently.
Addressing Alarm Fatigue
Alarm fatigue is a pervasive issue in clinical settings, where nurses are inundated with non-urgent or false alarms from monitoring devices. This phenomenon not only contributes to stress and burnout among nursing staff but also risks desensitization to critical alarms, potentially jeopardizing patient safety. AI systems mitigate this problem by employing advanced algorithms to analyze the urgency and context of alarms. For instance, machine learning models can differentiate between routine fluctuations and clinically significant anomalies, ensuring that nurses are only notified when necessary.
Predictive Analytics for Early Intervention
Predictive analytics, powered by AI, is another transformative development in patient monitoring. These tools use historical and real-time data to identify patterns that indicate the early onset of complications. For instance, machine learning models can predict sepsis—a leading cause of hospital mortality—hours before symptoms become evident. Early detection systems, such as Epic Sepsis Model, use algorithms trained on large datasets to calculate risk scores for patients. Research by Wong et al. (2019) found that such systems improved survival rates by enabling early administration of antibiotics and other interventions. Nurses play a pivotal role in interpreting these predictions and coordinating timely responses, making the integration of AI into monitoring workflows indispensable.
2. Streamlining Data Analysis in Clinical Settings
Simplifying Data Interpretation
The volume of data generated in modern healthcare settings is immense, with each patient generating hundreds of data points daily through diagnostic tests, monitoring devices, and EHRs. Nurses often face the daunting task of synthesizing this information to inform clinical decisions. AI tools, particularly those employing natural language processing (NLP), are transforming this process by extracting actionable insights from complex datasets.
For example, NLP algorithms can analyze unstructured data, such as physician notes, lab results, and imaging reports, to identify trends and highlight abnormalities. Topaz et al. (2020) reported that these tools significantly reduced the cognitive load on nurses, allowing them to prioritize high-risk patients effectively. Moreover, AI-driven dashboards provide visual summaries of patient data, enabling quick and accurate assessments.
Supporting Evidence-Based Decision Making
AI-powered Clinical decision support systems (CDSS) are increasingly integrated into EHR platforms, assisting nurses in clinical decision-making. These systems analyze patient data in real time and provide evidence-based recommendations for interventions, dosage adjustments, and care pathways. For instance, a CDSS might recommend adjustments to a patient’s insulin regimen based on glucose monitoring trends. By reducing uncertainty and ensuring adherence to clinical guidelines, these tools empower nurses to deliver high-quality care.
Fostering Interdisciplinary Collaboration
AI also facilitates interdisciplinary collaboration by centralizing and standardizing patient data. Nurses, physicians, and other healthcare providers can access unified dashboards that integrate AI-generated insights, promoting coordinated care. This is particularly beneficial in complex cases, where timely communication and data sharing are critical.
3. Reducing Administrative Burden
Automation of Routine Tasks
Administrative tasks, such as scheduling, documentation, and inventory management, consume a significant portion of nurses’ time. AI is alleviating this burden by automating repetitive tasks, thereby freeing nurses to focus on patient care. For example, AI-driven scheduling tools, like Kronos, optimize staff allocation based on patient census, anticipated workload, and staff availability. Carroll et al. (2022) found that these systems improved nurse satisfaction by reducing scheduling conflicts and ensuring equitable workload distribution.
Similarly, AI-powered inventory management systems track the usage of medical supplies and automatically generate restocking orders, reducing the time nurses spend on procurement tasks. These innovations enhance operational efficiency and ensure that resources are always available when needed.
Streamlining Documentation
Documentation is a cornerstone of nursing practice, but it is often time-consuming and prone to errors. AI-driven voice recognition tools, such as Dragon Medical One, allow nurses to dictate patient notes directly into EHRs. Lin et al. (2020) reported that this technology reduced documentation time by 30%, enabling nurses to spend more time on direct patient care. Additionally, AI algorithms can populate EHR templates automatically by extracting relevant information from monitoring devices and lab results, further simplifying the process.
4. Ethical and Practical Challenges
Data Privacy and Security
The integration of AI into nursing workflows raises significant concerns about data privacy and security. Patient data, often collected and analyzed by third-party AI platforms, must be protected from breaches and unauthorized access. Ensuring compliance with regulations such as the Health Insurance Portability and Accountability Act (HIPAA) is critical. Nurses must also be educated on data-sharing practices to uphold patient confidentiality.
Algorithmic Bias
AI algorithms are only as reliable as the data on which they are trained. Bias in training datasets can lead to inequitable outcomes, particularly for underrepresented populations. For example, predictive models that fail to account for socioeconomic or racial disparities may misclassify risk levels, leading to suboptimal care. Addressing these biases requires ongoing scrutiny and the inclusion of diverse datasets during algorithm development.
The Need for Training
As AI becomes integral to nursing workflows, it is essential to equip nurses with the skills to use these technologies effectively. This includes training on interpreting AI-generated insights, troubleshooting errors, and understanding the limitations of algorithms. Clarke et al. (2021) emphasized the importance of integrating AI education into nursing curricula to prepare the workforce for this transition.
References
- Carroll, J. K., et al. (2022). Optimizing nurse staffing through AI-powered scheduling systems. Journal of Healthcare Management, 67(4), 456-463.
- Clarke, D., et al. (2021). Addressing ethical challenges in AI adoption for nursing. Nursing Ethics, 28(5), 642-654.
- Lin, S., et al. (2020). The impact of voice recognition technology on nursing documentation. Healthcare Informatics Research, 26(3), 189-198.
- Ruppel, H., et al. (2021). EarlySense monitoring system: Reducing alarm fatigue and improving patient outcomes. Critical Care Medicine, 49(2), e165-e171.
- Topaz, M., et al. (2020). Natural language processing in nursing: Transforming patient care. Nursing Research, 69(1), 40-48.
- Wong, A., et al. (2019). Early sepsis detection with machine learning. Critical Care, 23(1), 187.