Book Appointment Now
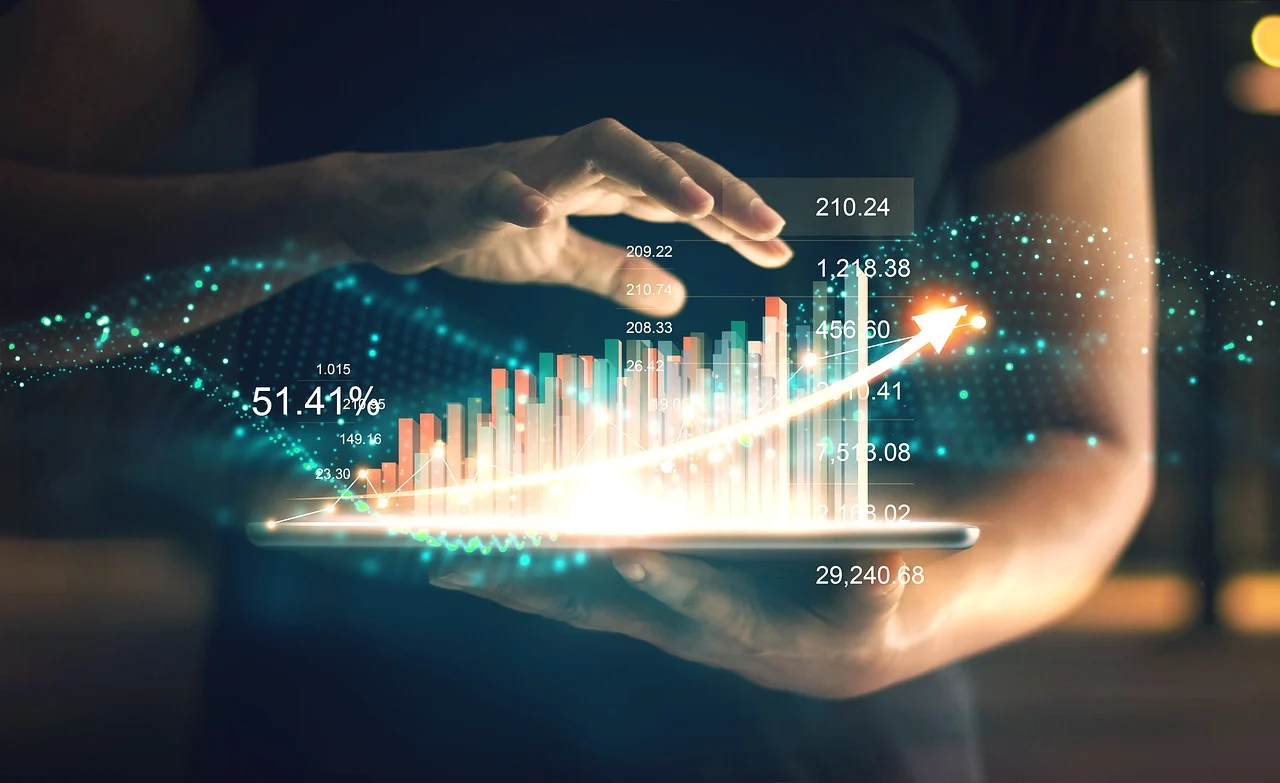
Understanding Predictive Analytics in Nursing
Predictive analytics in nursing has transformed how healthcare providers anticipate and respond to patient needs. By leveraging predictive models, nurses and clinical staff can proactively address potential health complications and risks, leading to better outcomes, reduced readmission rates, and overall improved patient care. This data-driven approach empowers healthcare professionals to make informed decisions that align with each patient’s unique needs, ultimately enhancing the quality of care.
Get a custom nursing paper help with your predictive analytics in nursing assignment
Order Custom Nursing Paper
How Predictive Models Identify At-Risk Patients
Through predictive models in healthcare, nursing teams can evaluate extensive patient data to recognize patterns and identify those at higher risk for complications. Predictive algorithms assess key factors, including medical history, current vitals, demographics, and lifestyle, to provide an accurate picture of a patient’s health trajectory. For example, these models can help identify patients likely to develop conditions such as sepsis, respiratory issues, or complications post-surgery.
By pinpointing these high-risk individuals, nurses can deliver proactive nursing interventions that reduce the likelihood of complications. With this approach, healthcare providers shift from reactive care to preventive care, directly impacting patient safety and health outcomes.
Proactive Care Interventions Using Predictive Analytics in Nursing
Once a patient is flagged as high-risk, predictive analytics in nursing allows for a range of proactive interventions that can prevent complications. Here are some common strategies enabled by predictive insights:
- Enhanced Monitoring: Patients identified as high-risk are monitored more closely, allowing for early intervention if symptoms worsen.
- Personalized Treatment Adjustments: Predictive data enables healthcare providers to adjust treatment plans to align with each patient’s specific needs and risk factors.
- Resource Allocation: Predictive analytics helps nursing teams allocate resources more effectively, such as assigning additional staff to high-risk patients.
- Preventative Education: Nurses can provide targeted education to patients on lifestyle and preventive care measures that lower the risk of readmission.
These interventions foster a care environment that emphasizes prevention, helping patients maintain health and avoid the costly and often traumatic experience of complications or hospital readmissions.
Benefits of Predictive Analytics in Nursing for Patient Outcomes
The adoption of predictive analytics in healthcare delivers numerous advantages for patient care, reducing risks and enhancing clinical outcomes. Here are some key benefits:
- Reduced Readmissions: By identifying risk factors early, predictive analytics aids in decreasing readmission rates, especially for chronic conditions such as heart disease, diabetes, and respiratory illnesses.
- Optimized Care Delivery: Nurses can offer timely interventions based on predictive insights, ensuring that high-risk patients receive prioritized, attentive care.
- Improved Patient Satisfaction: Preventing complications enhances patient experiences, as they receive care tailored to their needs and are empowered to take preventive actions.
- Cost Savings: Proactive care is often less expensive than treating complications or readmissions, reducing the overall cost of healthcare.
Real-World Applications of Predictive Analytics in Nursing
Healthcare organizations across the globe are leveraging predictive analytics to anticipate patient needs and mitigate risks. Examples include:
- Sepsis Prevention: Predictive models analyze EHR data to detect early signs of sepsis, enabling nurses to intervene before the condition becomes critical.
- Fall Risk Prediction: Algorithms assess factors like age, medications, and mobility to predict which patients are at higher risk of falling, leading to tailored preventive measures.
- Readmission Risk Reduction: For post-operative or chronically ill patients, predictive analytics identifies those at high risk for readmission, allowing healthcare providers to implement post-discharge care plans that lower this risk.
Using Predictive Healthcare Tools for Better Decision-Making
Tools like Electronic Health Records (EHRs) and Clinical Decision Support Systems (CDSS) integrate predictive analytics, making them valuable assets in daily nursing practice. By providing real-time alerts and recommendations, these tools guide nurses to make data-backed decisions that directly impact patient care quality.
For example, a predictive model may alert a nurse to a patient’s potential risk for adverse drug reactions based on their current medications and health conditions. With this information, the nurse can take immediate action, adjusting the treatment plan or consulting with a physician to minimize the risk.
The Future of Predictive Analytics in Nursing
As the healthcare industry advances, predictive analytics in nursing is poised to become an even more integral part of patient care. Future developments in artificial intelligence and machine learning are expected to make these models more precise, enabling even earlier identification of potential risks. With the continued integration of these technologies, nursing teams will be equipped to anticipate needs with greater accuracy, ultimately supporting a more proactive and preventive approach to healthcare.
Predictive analytics in nursing is a powerful tool that enables healthcare providers to anticipate patient needs and proactively manage risks. By harnessing predictive models, nursing teams can deliver personalized, preventive care that enhances patient safety, reduces readmissions, and optimizes healthcare outcomes. As predictive analytics continues to evolve, its role in shaping a data-driven future for healthcare remains invaluable.